WHITE PAPER
A Simple Explanation of How AI Can Be Used in Insurance
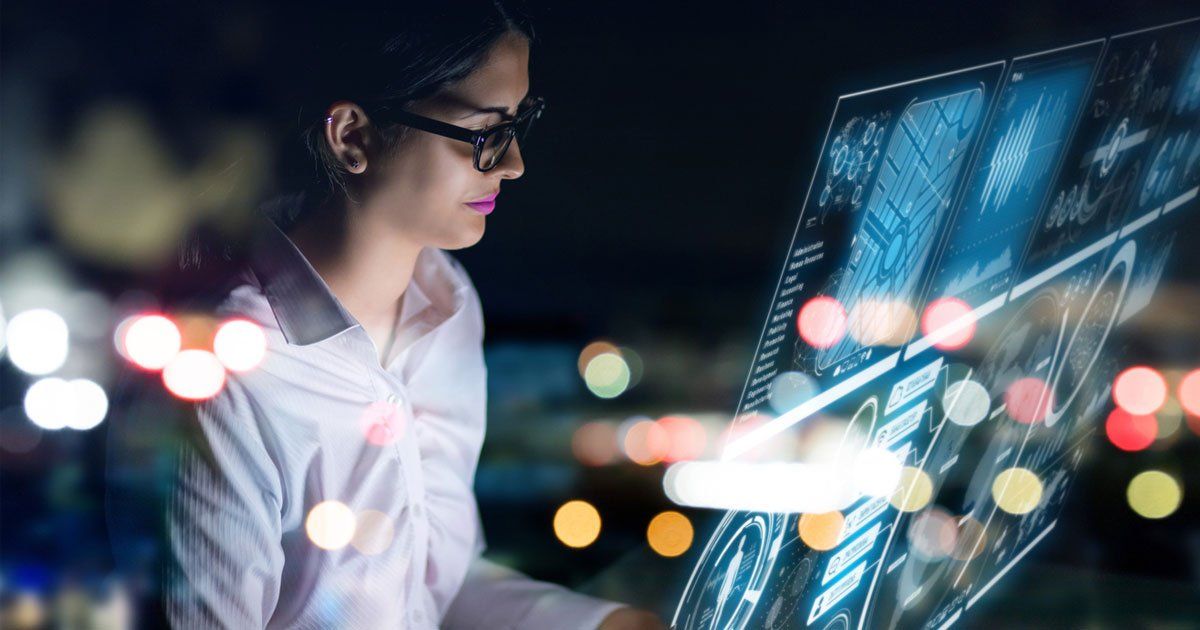
AI in Insurance: Practice Makes Perfect
As the saying goes, “practice makes perfect.” In other words, people learn from experience. According to the Oxford Dictionary, “regular exercise of an activity or skill is the way to become proficient in it, especially when encouraging someone to become persistent in it.” While practice cannot truly make someone perfect in any industry or activity, persistence does usually lead to increased proficiency, and seasoned practitioners typically outperform those who are just starting out.
In the insurance world, this concept applies as well, for both underwriting and claims management. A new underwriter gets better at predicting the cost of a new policy by seeing more policy applications, evaluating them, pricing them, and then seeing whether the price quoted results in a favorable or unfavorable loss ratio at the end of the policy term. Similarly, a new claims manager gets better at identifying and managing high-cost claims by seeing more new claims, triaging them, managing them, and then seeing whether the ultimate incurred is more or less than the amount of the reserve set.
Data science leverages this concept of repetitive practice leading to improved performance in the form of Artificial Intelligence or “AI.” At a high level, AI replicates the kind of human learning described above, but an AI model can “learn” from far more policies and claims than any person could ever hope to.
Where an experienced underwriter might see tens of thousands of applications in a career, an AI model can consider tens of millions of expired policies and the closed claims made against those policies. Further, the AI model can “remember” every fact in every one of those policies and claims and can compare those facts to every fact in every other policy and claim it’s “seen.”
This training process provides the model with the “knowledge” it needs to assess new underwriting applications and claims going forward, providing the organization with powerful capabilities to enhance its traditional operating practices.
Complicated Science; Understandable Principles
Understandably, before an insurance organization can adopt AI – or any technology – it must be understood before it can be implemented, and its value fully realized. While the science behind AI itself is complicated, the principles are not and can be understood easily with a few explanations and illustrations.
Just like a person learning to predict what’s going to happen when presented with a new set of facts, an AI model looks at an application or claim and takes stock of what characteristics make it similar to, and different from, all the policies or claims it’s seen in past.
Unlike a person, however, whose experience may be limited or biased, the model then evaluates those similarities and differences using a purely mathematical algorithm to predict what’s going to happen and determines the probability of a similar outcome.
Measuring the Accuracy of AI Models
To assess the model’s ability to predict the outcomes of policies or claims that it hasn’t seen in training, it first needs to be tested. This is typically done by showing it the facts presented in expired policies or closed claims without showing it the outcomes. That is, without showing it the claims actually made against those policies or the actual costs of those claims. The model is then asked to predict outcomes, and those predictions are measured against the actual outcomes.
AI replicates human learning, but an AI model can ‘learn’ from far more policies and claims than any person could ever hope to.
One way of measuring the accuracy of the model is to compare its performance against a random distribution. For example, if one million claims are randomly distributed into five buckets, the average cost of the claims in each bucket would be very close to the same – each bucket would have a lot of smaller claims, a few larger ones, and handful of mega- claims, but the average cost of the claims in each bucket would be about the same. The distribution, often illustrated with a bar chart, would look like this:
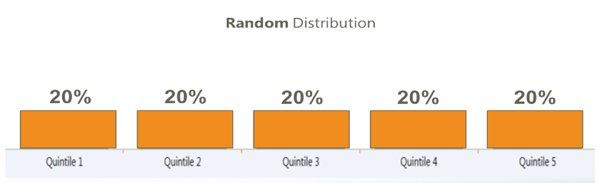
If, however, the model is asked to predict the claims that are least likely to be expensive and put those in the bucket on the left, and the claims that are most likely to be most expensive and put those in the bucket on the right, and to distribute the rest of the claims along that range, the bar chart should look like some version of this:
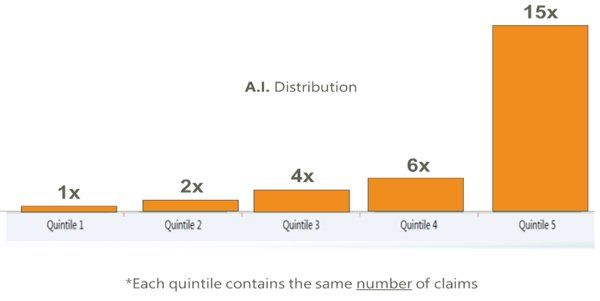
In this example, the model can accurately distinguish between the low-cost claims on the left and the high-cost claims on the right. The difference in the average cost of the claims in each bucket is known as “lift”. The model was not only able to predict the highs and the lows, but also to predict everything in between in the correct general order – this is known as “linearity”, and the predictions of a good model should be linear in this sense.
How is AI Relevant to Your Business?
So why should you care about AI and what is its relevance your business? By leveraging AI and machine learning, insurers of all types are realizing important operational and business benefits that include:
Underwriting
- Pricing policies more accurately to reflect the risk they represent
- Reducing quote turnaround times to capture more business
Claims
- Triaging claims to ensure that those with highest potential costs get the attention they deserve earlier in the life cycle, starting at first notice of loss
- Reducing the duration and cost of claims
This a simple explanation of the vast potential for AI in the insurance enterprise. For additional examples of how these models can be used to improve your company’s underwriting and claims processes, visit our resources page gradientai.com/resources.
About Gradient
Gradient AI helps insurers of all types achieve a better return on risk. A leading provider of proven artificial intelligence (AI) solutions purpose-built for the insurance industry, Gradient improves loss ratios and profitability by predicting underwriting and claim risks with greater accuracy, as well as reducing quote turnaround times and claim expenses.
Unlike other providers that use a limited claims and underwriting dataset, Gradient’s software-as-a service (SaaS) platform leverages a vast dataset comprised of tens of millions of policies and claims. It also incorporates numerous other features including economic, health, geographic, and demographic information. Customers include some of the most recognized insurance carriers, MGAs, TPAs, risk pools, PEOs, and large self-insureds across all major lines of insurance.
To learn more about Gradient, contact us.