Beyond Big Data: Why Quality Data Matters in Workers’ Comp AI Models
May 15, 2025
By Stan Smith
For insurance executives evaluating AI solutions, here’s the critical truth: Your AI is only as good as your data. In workers’ compensation, where medical and legal complexities are especially prevalent, quality well-structured quality data separates market leaders from the rest. Underwriters and claims professionals require high-quality data to make informed decisions that reduce risk, control costs, and improve outcomes. As AI plays a larger role in underwriting and claims management, the need for reliable, comprehensive data has never been greater.
Strengthening Workers’ Compensation Underwriting
In workers’ compensation as well as other types of insurance coverage, accurately assessing a group’s risks ensures competitive and sustainable pricing commensurate with the group’s risk. But underwriting is only as good as the data it’s built on.
AI-powered work comp underwriting models need vast amounts of data covering every class code, region, and risk factor. The broader and more representative the data, the better insurers can account for industry-specific risks, regional differences, and emerging trends. Without a dataset that is both broad and deep, AI models may not assess risk accurately—potentially leading to underpriced policies that negatively impact insurers’ loss ratios, or overpriced ones that drive businesses away. Similarly, insurers looking to expand into new geographies or lines of business rely heavily on extensive quality data to help them assess risks in these new markets.
Reliable data is critical for fueling predictive analytics, which helps insurers anticipate workplace injury risks based on historical claims, job conditions, and even economic trends. This foresight lets insurers work with employers to put risk prevention strategies in place-ultimately reducing the frequency and severity of claims.
Improving Claims Management
Quality data also supports claims management. When a workplace injury occurs, insurers must quickly determine the best course of action. AI-driven claims analysis, powered by vast datasets and insights from previous claims, helps insurers recognize patterns that indicate whether a claim is likely to escalate, require litigation, or benefit from an independent medical exam (IME). A study by Travelers found that first-year employees account for 35% of workplace injuries, leading to over 6 million lost workdays.
AI models trained on historical claims data can flag high-risk cases early, allowing claims managers to intervene proactively to help minimize claim duration and cost. If certain injuries in a specific industry tend to lead to extended time off, claims adjusters can implement early return-to-work programs tailored to those cases.
Access to quality data also helps uncover fraud. The Coalition Against Insurance Fraud estimates that fraudulent claims cost the industry over $308 billion annually. AI-powered fraud detection tools analyze claims histories, provider patterns, and inconsistencies in reported injuries to identify red flags early helping insurers take action before costs spiral out of control.
Why High-Quality, Reliable Data Matters
AI models are only as effective as the data they rely on. If data is incomplete, outdated, or inconsistent, predictions become unreliable, leading to flawed risk assessments and poor decision-making. An insurer my company works with recently found that 40% of its most critical data fields were missing or incomplete, making it difficult to build reliable AI models. This issue is widespread—missing or inconsistent data limits an organization’s ability to identify patterns and make informed decisions. AI thrives on detail, whether it’s analyzing claims demographics or tracking operational risk trends, and yet many insurers face similar problems with “messy” data. However, without complete and standardized data, AI models can’t generate accurate insights.
Digital Insurance highlights that top-performing insurers set themselves apart by prioritizing rigorous data validation and reconciliation processes.
To fully harness AI-driven insights, insurers need data that is:
- Accurate: Free of errors to support sound risk evaluations and claims decisions.
- Comprehensive: Captures all relevant details about policyholders, claims, and medical treatments.
- Consistent: Standardized across different sources to prevent discrepancies.
- Timely: Continuously updated to reflect real-world risks and conditions.
- Unique: Avoids duplicate or conflicting records that could distort insights.
Fortunately, insurers do not need to rely solely upon their own databases to implement AI effectively. A well-structured contributory data lake, which aggregates anonymized claims data from multiple insurers, offers a wider view of industry risk trends. This shared intelligence allows insurers to benchmark their claims experience against broader industry patterns, leading to more accurate underwriting and more effective claims management strategies. Insurers can leverage such a data lake without necessarily addressing their own data hygiene, and access a far larger and higher quality dataset than they ever could within their own four walls.
Steps to Strengthen Data for AI
To maximize AI’s potential, insurers must proactively enhance their data environments.
Steps include:
- Assessing data quality: Conduct a data census to evaluate completeness, consistency, and accessibility.
- Identifying gaps and clean data: Fill missing fields by integrating external sources or using tools to infer incomplete information.
- Establishing governance policies: Implement standardized data collection methods, such as mandatory fields, dropdown menus, and validation checks.
- Checking for bias: Analyze datasets to ensure fairness across demographic and geographic segments, reducing skewed AI outcomes.
By implementing these strategies, insurers create a strong foundation for AI-powered decision-making, ensuring models operate with accuracy and fairness.
Measuring AI Success in Workers’ Compensation
Once an AI-driven approach is in place, insurers need clear ways to track its effectiveness. Key methods include:
- Defining success metrics: Establish measurable goals, such as improved claims processing speed or risk assessment accuracy.
- Backtesting models: Validate AI predictions by applying them to past claims data and comparing results to actual outcomes.
- A/B testing: Compare AI-driven processes with traditional approaches to determine effectiveness in real-world scenarios.
By consistently measuring and refining AI performance, insurers can ensure their models continue to drive better underwriting, claims management, and fraud prevention.
The Future of AI in Workers’ Compensation
AI is reshaping workers’ compensation, but its success hinges on the quality and depth of data. Insurers that invest in strong data strategies will gain a competitive edge, developing pricing models that accurately reflect risk, improving claims decision-making, and proactively identifying high-risk claims. Enhanced fraud detection will also help reduce unnecessary payouts, strengthening industry efficiency.
Ultimately, insurers that prioritize high-quality, well-structured datasets will lead the way in delivering fairer, more efficient, and cost-effective workers’ compensation solutions. AI is a powerful tool, but its success hinges on the strength of the data behind it.
Stan Smith is founder and CEO of Gradient AI and has nearly 30 years of experience in AI and technology. Drawing on the latest advancements in AI and machine learning, he launched Gradient AI to help insurers harness data more effectively. The company’s solutions empower insurers to enhance underwriting accuracy, streamline claims processes, and ultimately improve loss ratios and profitability.
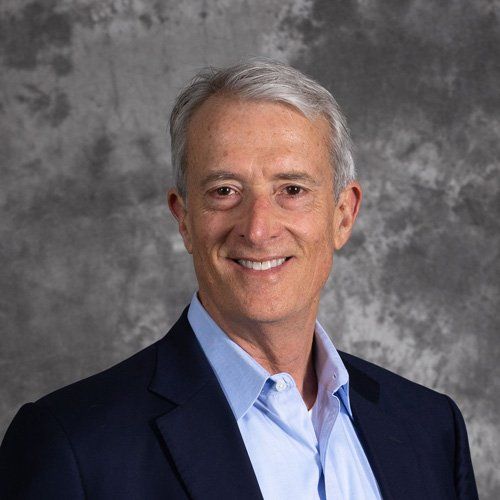
Stan Smith, Gradient AI
Stan Smith is founder and CEO of Gradient AI and has nearly 30 years of experience in AI and technology. Drawing on the latest advancements in AI and machine learning, he launched Gradient AI to help insurers harness data more effectively. The company’s solutions empower insurers to enhance underwriting accuracy, streamline claims processes, and ultimately improve loss ratios and profitability.
This article first appeared on
WorkCompWire.